Issue #18 – Organisational Structures for Today's Data Realities
Data has become harder because normal org structures don’t work
Read time: 12 minutes
One would think that the org structure for the data world should be pretty straightforward.
You have a data team. They sit in their own function (or with IT sometimes) and are set up to interact with other departments as per the business’s requirements.
If only it were that easy…
Fitting data into an organisation’s structure is proving more complicated than most companies can handle.
Why the Org Structure is So Important, Yet Hard to Do
Establishing the right organisational structure is critical for any company. The structure underpins collaboration, demonstrates how data teams work across the organisation, and ultimately determines how well the company can leverage data to drive business outcomes. A well-designed organisational structure can enhance efficiency, foster innovation, and ensure that data initiatives align with business goals.
However, creating such a structure is often challenging.
Organisations must balance competing priorities, manage interdepartmental dependencies, and adapt to evolving business needs and technological advancements.
I’ve broken down the difficulties of setting up the ‘right’ organisational structure into five reasons. I also want to mention that most organisations have poorly designed overall organisational structures for other functions/ the whole company, so these themes aren’t contained to data specifically:
Ownership – The data team is cross-functional in nature, creating inherent ownership issues. Data needs to interact with every department within the company to be beneficial. For some organisations, that means having data scientists or analysts on the finance or marketing team. For others, it means a centralised data team with dotted lines to other departments. This cross-functional nature of data and analytics makes it hard to nail down that ownership, which may become easier in other departments and domains.
Team Evolution – Data teams and their remit are evolving quickly. Finding the best structure is a hard task for a static team. Now imagine a team adding new roles or teams every few months, roles that may not have existed before or been properly defined. It's impossible! What you get is an HR employee searching for answers on ChatGPT about where new employees should sit, who they should report to, and what their responsibilities should be.
Ill-Defined Role – Speaking of misunderstanding roles, data’s role in the business is still poorly understood. It is impossible to properly integrate a new team into an existing organisational structure if you don’t know what that team is there to accomplish. A business executive’s initial perspective on data usually extends to news stories about Machine Learning, AI or new privacy regulations. After some homework, they might learn about BI dashboards, data engineering, and data governance, but learning the capabilities is not the same as understanding them.
Data Leadership Vacuum – Most organisations have a vacuum in data leadership. Teams need leaders to succeed. If the data industry is missing one thing, it is good leaders. Your best data person might be a technical genius but probably doesn’t have the communication skills to hold a ten-minute conversation with the CEO. The highest-ranking data person rarely gets invited to the top table, especially given that only 27% of leading global organisations have a CDO. All said, without a data leader pushing for the ideal structure, the data team will often get placed in two or three different places in different departments, limiting their impact.
Corporate Politics – Nobody talks about this, but corporate politics is the single biggest hindrance to an organisation making progress in its data capability. No organisation exists without politics, and nothing is more political than people’s jobs. Every single org structure change can very easily get political, whether it is hiring, firing, changing reporting lines, revising responsibilities or moving teams. And the negative politics builds on all the reasons we went through above.
These are five reasons why embedding data into an organisational structure is hard. More exist, and these reasons also extend to other negative outcomes (like lack of ownership, poor data literacy and skills, or siloed teams).
How to Approach the Right Data Org Structure
Before defining the different approaches to a data org structure, it is important to consider how to approach choosing the right one for your organisation. This depends on several factors:
Business Goals – Aligning the data strategy and org structure with overarching business objectives.
Maturity of Data Capabilities – Assess the current state of data expertise, infrastructure operations and ability to deliver on data activities/ initiatives
Culture and Leadership – How mature is the data culture? Is the organisation being led by executives who understand the value of data?
Cross-Departmental Collaboration – Understanding the level of collaboration between functional business departments and data teams today
Simply put, a one-size-fits-all approach doesn't work. Some may benefit from a centralised model, while others might need a more decentralised approach, but every organisation will have a unique approach that goes beyond theory.
So, as you read the next section, think about those four components!
Overview of the Three Generic Org Structures
There are three primary types of organisational structures for data teams: centralised, decentralised/mesh, and hybrid (hub & spoke).
Each has its strengths and weaknesses. Each fits better into some industries than others. Each has evangelists in the data world who clamour for it to be the best pick.
That being said, every organisation tends to have a unique hybrid approach. Instead of looking at this like three distinct approaches, look at it as a spectrum. Some teams will be centralised through and through, while others might decentralise teams based on functions or regions, but have a Centre of Excellence (CoE) for certain data capabilities.
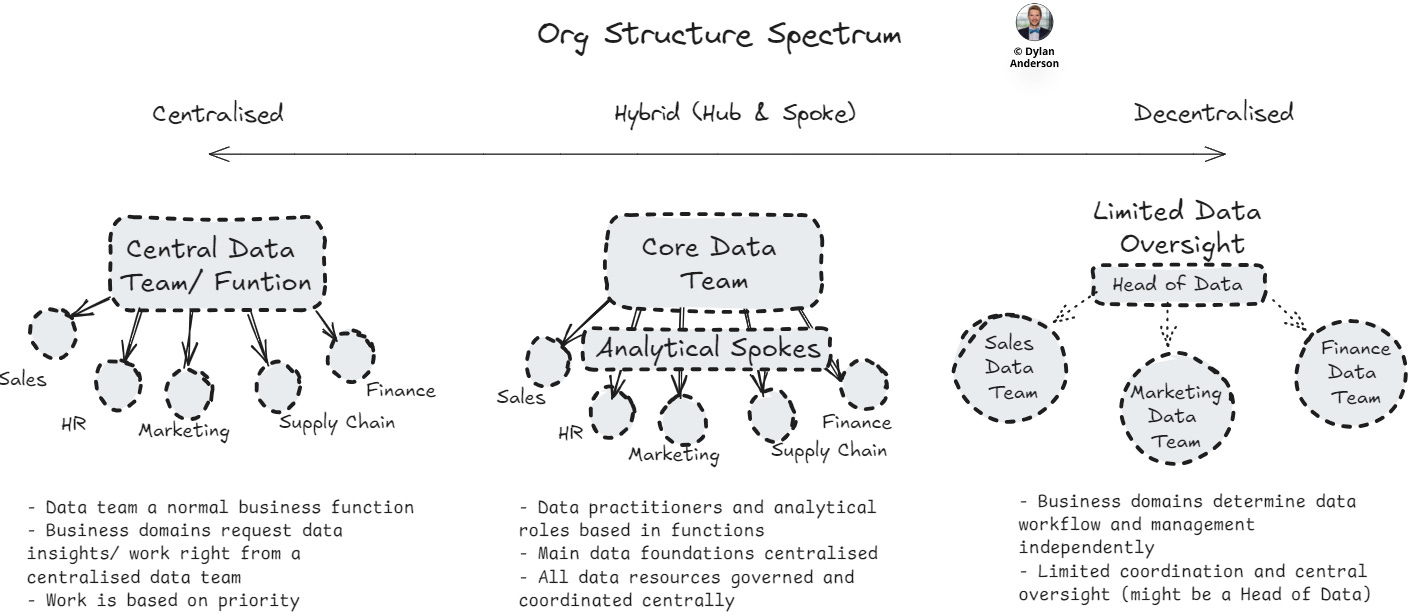
Centralised Structure
The centralised structure is the most basic view of data where all data functions are consolidated within a single, central team or department. They are responsible for all data activities, from storage to processing & transformations to distributing insights. Usually, companies start with this type of structure, given its simplicity and the need to have all the data operations in one place.
Pros:
Consistent data governance and standards
Easier to maintain data quality and security within the platform and across analytical tools
Best practice is easily disseminated between team members
Efficient resource allocation and simplified communications within the team
Clear accountability within the data team
Cons:
Can create bottlenecks in data access and processing
May be less responsive to individual department needs
Lack of business context within data initiatives and teams
Difficult to scale within a large, distributed organisation
Best Fit: Smaller and medium organisations who are just getting started on their data journey, especially those with straightforward data needs or in highly regulated industries requiring strict data controls/ ownership
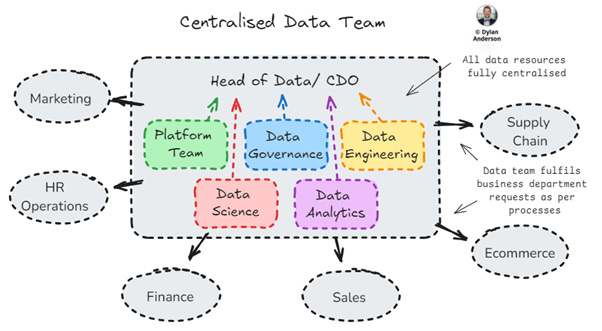
Decentralised/Mesh Structure
A decentralised data structure, often implemented as (or called) a data mesh, distributes data ownership, management, and activities across various domain-specific teams within the organisation. Each team is responsible for building its own data products and solutions and managing all other data capabilities within that. Companies take a decentralised approach to develop functional domain experience (e.g., marketing, finance) within data teams and to build business-relevant products in a more agile way without the bureaucracy that centralised teams can bring.
Pros:
Increased agility and responsiveness to specific business needs
Closer ties between business stakeholders and domain data teams
Should increase data literacy within functional domains given close exposure to managing their data and building products/ solutions
Easier to scale in complex, large organisations
Promotes innovation, customisation and ownership
Cons:
Can lead to data silos and inconsistencies, especially in data platform development, engineering and governance
Requires strong coordination and collaboration to do effectively
Often duplication of effort or lack of sharing best practice across teams
Can lead to paying for multiple tools that do the same thing
Challenging to implement and govern effectively
Best Fit: Suitable for large, complex organizations with diverse data needs, such as multinational corporations or companies with varied product lines. Better suited for more mature data teams who have processes to encourage cross-functional collaboration across core data capabilities (e.g., engineering, governance, analytics, etc.)
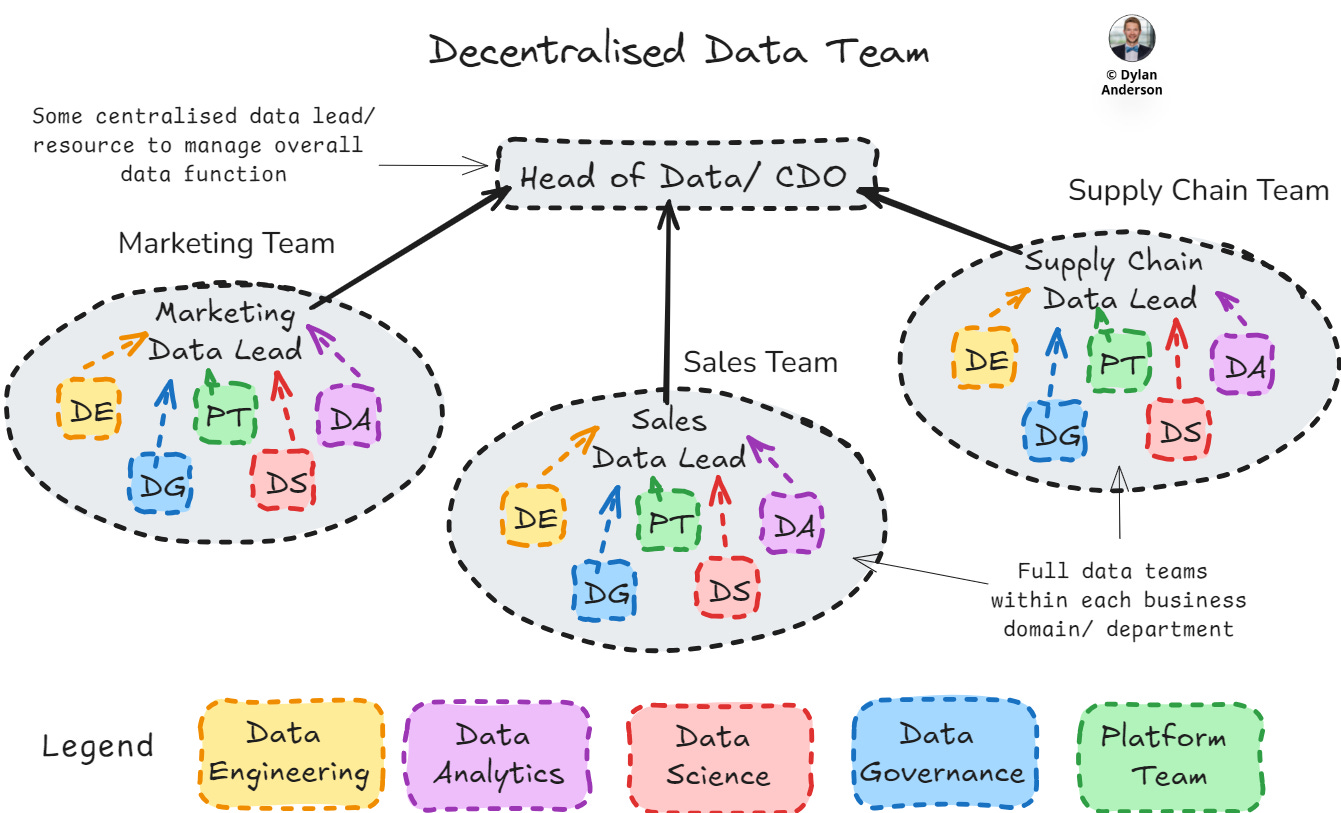
Hybrid/Hub & Spoke Structure
The hybrid approach (or hub-and-spoke model) is the most common organizational structure due to the abovementioned spectrum point. A hybrid org structure combines elements of both centralized and decentralized structures, usually having a central hub for infrastructure and governance with spokes (individual business units) managing domain-specific data activities like analytics.
Pros:
Focuses on balancing consistency across infrastructure/ governance with flexibility when it comes to data solutions
Easier standardisation of best practice and core data elements
Provides closer access to business stakeholders to meet their needs
Enhances collaboration and data sharing between central and business-specific data teams
Cons:
Complex structure that can be hard to implement and manage
Requires clear roles and responsibilities and strong processes to manage hub and spokes
Conflicting direction between hub and spokes
Consistency is challenged by ad hoc, agile activities within spokes
Needs strong data leadership to enable communication
Best Fit: Ideal for most small and large organisations, as companies often require central oversight with some diversification in product/ solution development. The hybrid philosophy also scales well in situations when companies transition from a pure centralised (e.g., team is growing) or decentralised (e.g., data got too siloed) approach
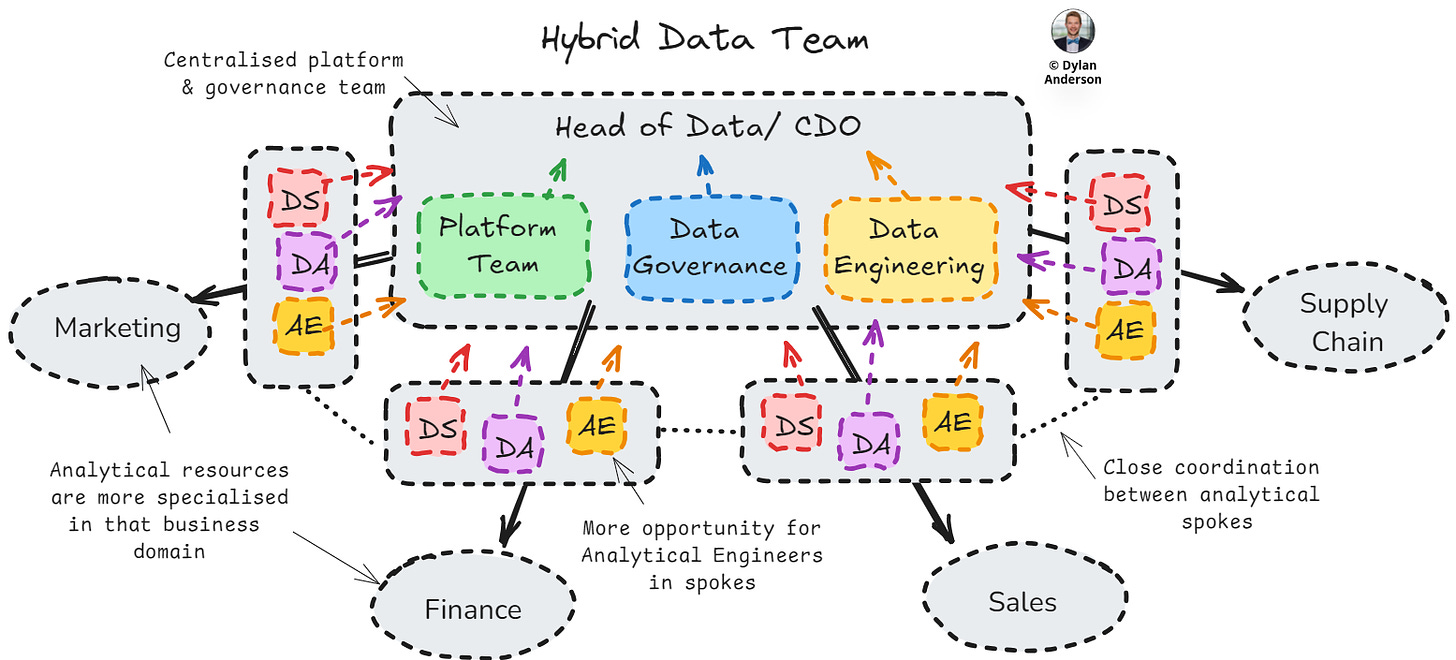
Building the Right Data-Enabling Org Structure
With all the context, the next step would be to design the right org structure for your organisation. There are a lot more considerations to make, but here is a basic approach that I’ve taken with multiple clients:
Define Strategic Objectives & Business Model – Start with the data strategy, business goals, and business model. Understand what the organisation aims to achieve with its data initiatives and how it is currently delivering against its strategy
Assess Current State – Map out how the organisation is currently set up (both the data and business functions). Understand how other comparable companies are doing it to provide a benchmark. Interview stakeholders to identify strengths, weaknesses, and gaps in the data capabilities and current structure
Design the Structure – Operate with a hypothesis-led approach and design a to-be organisational structure based on the assessment and strategic objectives. Define the roles and responsibilities of central and business unit data teams, refining where necessary through workshops with key stakeholders
Update Governance Structure & Processes – Identify how the new structure will impact business processes. Then, governance frameworks (e.g., workflows, RASCI) and forums will be established to support the chosen structure. Allow for flexibility within these to adapt as necessary
Define Change Management Approach – Set out the plan, including milestones, workshops, stakeholder touchpoints, and any logical sequencing to implementation. This can be done through communication channels, regular meetings, joining initiatives and tools that facilitate information sharing and alignment
Implement & Iterate – In line with the plan, implement the change. Set KPIs to monitor the progress of execution and effectiveness when in operation. Solicit feedback from data and business teams for improvement, allowing the evolution of the structure as the organisation grows and business/ data needs change
This approach ensures that the org structure is not just an afterthought of what exists but as an enabler to the overall business and data goals.
Final Considerations for the Org Structure
Before I conclude, there are three other considerations I want to mention:
The first is to think about how you can structure the data team to enable rather than exist. Many companies have vertically structured data teams next to other domains/ departments. The data team then ends up getting siloed because there isn’t the proper alignment with business teams. Therefore, I suggest setting up the data team horizontally across the business, with individuals linked to different business teams. This mimics a hybrid model and allows for close collaboration with your end users and core stakeholders.
The second consideration is the people, process, technology and data framework/ approach. I wrote about this at length last week, but the key to enabling your organisation to work well means taking a holistic approach with all four things in mind. Make sure each component helps influence the org structure design, especially processes (crucial to enable how teams work across the org structure) and people (which set the trajectory of the org structure design). Without this in mind, things fall through the cracks.
The third consideration is culture. The org structure is the single biggest enabler of a data-driven culture. Who people work with and how they are set up to work with them is the foundation for the right mentality when it comes to data. I will write more about this subject, but the important things to keep in mind are (1) structuring teams to deliver initiatives with common goals in mind; (2) showcasing and sharing those wins; (3) having the right executive sponsorship and leadership to create a positive culture; and (4) creating a data literate organisation.
While the org structure is often an afterthought for companies, this article demonstrated why it is essential. It underpins everything you do in data! Hence, continuing with the status quo because org structure design is hard is short-sighted and will lead to issues later.
Over the next two weeks, we will jump into the technology component of structuring a data team. First, we will look at developing an overarching data technology strategy, considering the processes and considerations needed to choose and implement the right technologies. We will look at the overall Technology landscape the week after and define most of the tools within the Data Ecosystem. Then a couple of weeks on the Data Platform, both defining what a Data Platform is and discussing how to design it effectively.
I look forward to digging into it with you all. Until then, thanks for reading, subscribing, liking, and commenting!
Thanks for the read! Comment below and share the newsletter/ issue if you think it is relevant! Feel free to also follow me on LinkedIn (very active) or Medium (not so active). See you amazing folks next week!