Issue #12 – The Three Biggest Data Problems Companies Face
Having consulted with dozens of large organizations, these are the most common root causes for data issues
Read Time: 9 minutes
Do you know why work always seems busy and companies are always fighting fires?
Because there are always problems that need solving.
Every business domain has its core problems. Marketing doesn’t know enough about their customers. Finance needs to find ways to save money. Supply chain needs to cut inefficiencies.
And what about data? What is at the top of mind for data professionals and executives?
Well there is a difference between what is top of mind and what are the biggest underlying problems. For example, data leaders may say their biggest problem is not getting enough value from their data teams.
While this is true, it is systematic of a multitude of root causes and tackling the value question will only complicate manners.
So this week, I will use my experience of consulting for dozens of large organisations and share with you the three biggest root cause problems that data companies are facing today.
Problem #1 – A Thrown Together Data Operating Model
One of the most widely used and referenced frameworks in data is the People, Process, and Technology framework (see below). Despite its popularity, organizations don’t do well using it for its desired purposes.
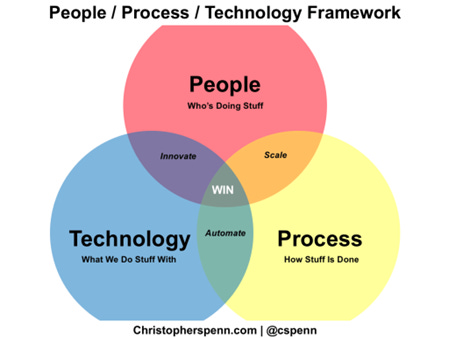
The purpose of the framework is to ensure all three elements are working symbiotically within the organization (i.e., the right people are supported by the right processes to enable the power of the right technological tools). The single biggest aid to deliver against this is to design an effective org structure and operating model.
Essentially what you see in most organizations is the data team set up as another vertical capability adjacent to all the other classic functions (e.g., marketing, finance, supply chain, etc.). The data People often sit outside the teams they support, siloed from being able to collaborate effectively. Moreover, sometimes these teams have quasi-data or analyst resources that fill the gap for data & analytics talent, but realistically they don’t have the right skillset (usually working from Excel) or the right direction and best practice.
And all while the data People sit siloed beside the capabilities they support, there are no official Processes to bridge that divide. Collaboration and coordination between data teams and business teams is usually based on informal relationships, resulting in data projects not being built with the business stakeholders in mind. Therefore, expensive third-party Technology or internally built data tools aren’t delivering the ROI as expected because nobody knows how to properly use them.
This comes down to no established ways of working, where the processes to bring people together and use technology effectively don’t exist. In the end, data effectiveness is the main casualty.
Let’s give an example. A popular new tool is a Data Catalogue, which allows business users to understand where data is and what it means. While this should create common ground between data and business teams, there needs to be coordination between the Data Governance and Analyst teams on the data side and the business users. A strategy to lay out the goals of the tooling, processes to fill out, update and use the tool, and initiatives to train users all need to be part of the implementation.
Instead, most companies buy the tool, don’t link together how the tool will be used across teams, offer disjointed training to business teams, and call it a day. The tooling fails because the operating model has not been considered.
One perspective I hold is that a horizontal type structure helps deal with all of this (after all, data supports each capability in the business). But to be effective, this has to be built in a way that still allows data people to work within a data team to ensure they learn and grow together. The most important piece is just setting up the right People with the right Processes to underpin any data activities, something most companies do not hit the mark on.
We will talk more about this subject next week, but now let’s get on to the next underlying problem!
Problem #2 – Lack of the Data Model Linkage
Data modelling has been around for a long time and used to be essential for companies to get right given the high cost of storage and computing resources and the nature of on-prem data warehouses. However, new data tools and technologies have deemed the practice less ‘popular’ because you don’t necessarily need it to achieve your data goals. That perspective is very focused on short-term thinking, which you see a lot in data.
To better explain the phenomena, here is a great quote from Keith Belanger on Joe Reis’s podcast about how data professionals and organisations are ignoring data modelling:
“The complaint I always hear, especially with the new crop of data practitioners, is data modelling seems very dated. It seems very slow and cumbersome and I have a job to do and I don't have time to learn all this stuff.
But we've never showed them or they've never really learnt on how to data model how to take a step back and understand. As I like to say, data modelling tells me a story, right? When you give me a data model, it's like reading a book. I can tell you about your organization.”
Anybody who has been in the data industry for more than a few years understands that to execute effectively, you need to consider the business, how it works and what it needs.
The data model is that connector!
Yet we keep building stuff without a data model to reference, and the more you build, the further the drift.
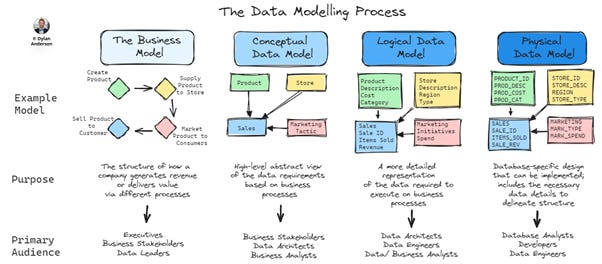
And unfortunately, as mentioned in my previous point, organizations are not set up to correct this drift. We focus on short-term fixes and incentives, like hiring multiple data engineers to build pipelines without the architectural foundation that a data model provides.
Overall, the speed at which we are working and trying to solve all these short-term problems (like data quality or building data outputs) makes us lose sight of the larger reasons why we are doing any of this in the first place. Not addressing a poor org structure or a lack of a data model is like constantly replacing buckets of water under a leaking roof without actually bothering to fix the leak.
The data modelling problem is a big one and something executives or data leaders don’t often pay attention to. We will talk at length about it in a couple of weeks.
Problem #3 – The Data Quality Question
Data quality is an output, not an input. But people don’t realise that.
It is a symptom of dozens of other problems you see within the data ecosystem. But fixing it on its own is not a single task or endeavour (unfortunately a lot of people think in this way). Instead, it needs to be approached from a multitude of angles.
But organizations don’t have that foresight. They want an immediate and simple answer to this problem, and I’ve never met a busy executive who likes the truth of it—that data quality needs a systemic, holistic approach across teams.
So, companies march on, and data quality issues continue to be pervasive, affecting everything from operational efficiency to strategic decision-making. They may try to fix quality through competent technology integrations, invest in a Data Catalogue, or hire data quality SWAT teams to manually fix identified issues.
All of these are partial solutions, but unfortunately, these only address surface-level causes, not the root causes. For example, poor data quality may come from:
Inconsistent data entry
Fragmented data systems
No standardized data definitions
Under-resourced data governance team
Lack of data owners & stewards in the business
No clear operating procedures for using data systems
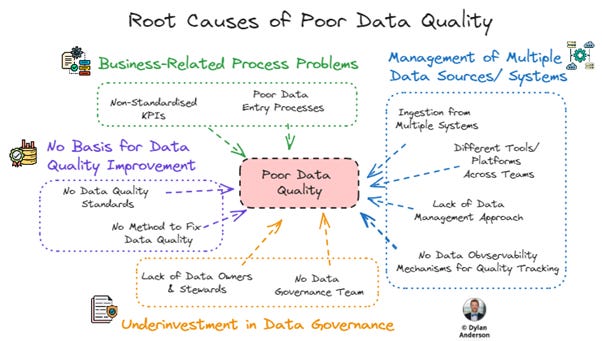
The first step is to identify which of these (and other) root causes are impacting data quality in your organization. This requires time, resources and taking a step back from the day-to-day firefighting, all of which are hard to come by in any organization. Interestingly, the two above problems (operating model and data modelling) will help solve this as well. Data Governance is an essential team to facilitate this discussion, set ownership, and begin to define data quality standards. The Data Model helps stakeholders understand the relationships between business processes and database contents, allowing data teams to define the importance and quality of different variables.
I should mention DAMA here. Data governance and quality professionals will reference the DAMA wheel to provide a guiding framework. While this is extremely helpful and DAMA is very knowledgeable in this space, the wheel is very academic. An organization needs to identify its own root causes and issues (potentially aided by these categories) that relate to how it operates. Then it can go solve for these things.
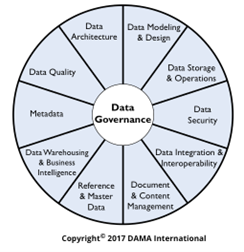
Given the size of the Data Quality problem, I will tackle this in more detail as well, so stay tuned!
Addressing the Big Three
In the end, every client I have, business I have witnessed and person I have engaged with can speak to having at least one of these three problems.
And the issue is, these problems lead to more problems:
Misaligned Data Operating Model – Poor ways of working with business teams; no value from data projects; siloed working; poorly perceived ROI from data spend
Lack of the Data Model Linkage – Constant engineering issues; no alignment between pipelines/ data products and analytical tooling; business context doesn’t translate to data foundations
No Holistic Approach to Data Quality – The business doesn’t trust the data; constant firefighting to improve data; lack of future data funding
While these three problems are pervasive (and extremely detrimental), there are solutions. Over the next three weeks I will be talking about each one from that perspective—how do we approach these issues in a realistic, non-academic way? I want to get the guts of how to start fixing these issues, so stay tuned as we tackle three of the most interesting topics in the Data Ecosystem!
Thanks for the read! Comment below and share the newsletter/ issue if you think it is relevant! Feel free to also follow me on LinkedIn (very active) or Medium (not so active). See you amazing folks next week!
Great Article. I work in a Higher Education industry where we buy the application for our needs and most of the time these applications are delivered with a very complex data model. That being said, the applications that are currently being written are service based and we don’t see a data model there for these services. The applications hosted on the cloud creates additional challenges. This creates a lot of headache for the data teams that are responsible for Datawarehouses and Operational Data Stores. All these applications that are bought and developed are creating data silos that the leaders are not aware of. If we are able to fix the issues at the top in the application chain, the downstream application like Datawarehouses and Operational Data store might not have to go through the headaches.
Great article! I am looking forward to keeping up with these posts. What I have seen recently from clients is they expect to throw the new shiny tool at the problem and their data quality woes will go away. However, as you have aptly pointed out in this article that data quality, failed data initiatives, etc. are a symptom of _many_ problems. From my experience, organizational leaders, for whatever reason don't seem to grasp the importance of stepping back and evaluating the problem.